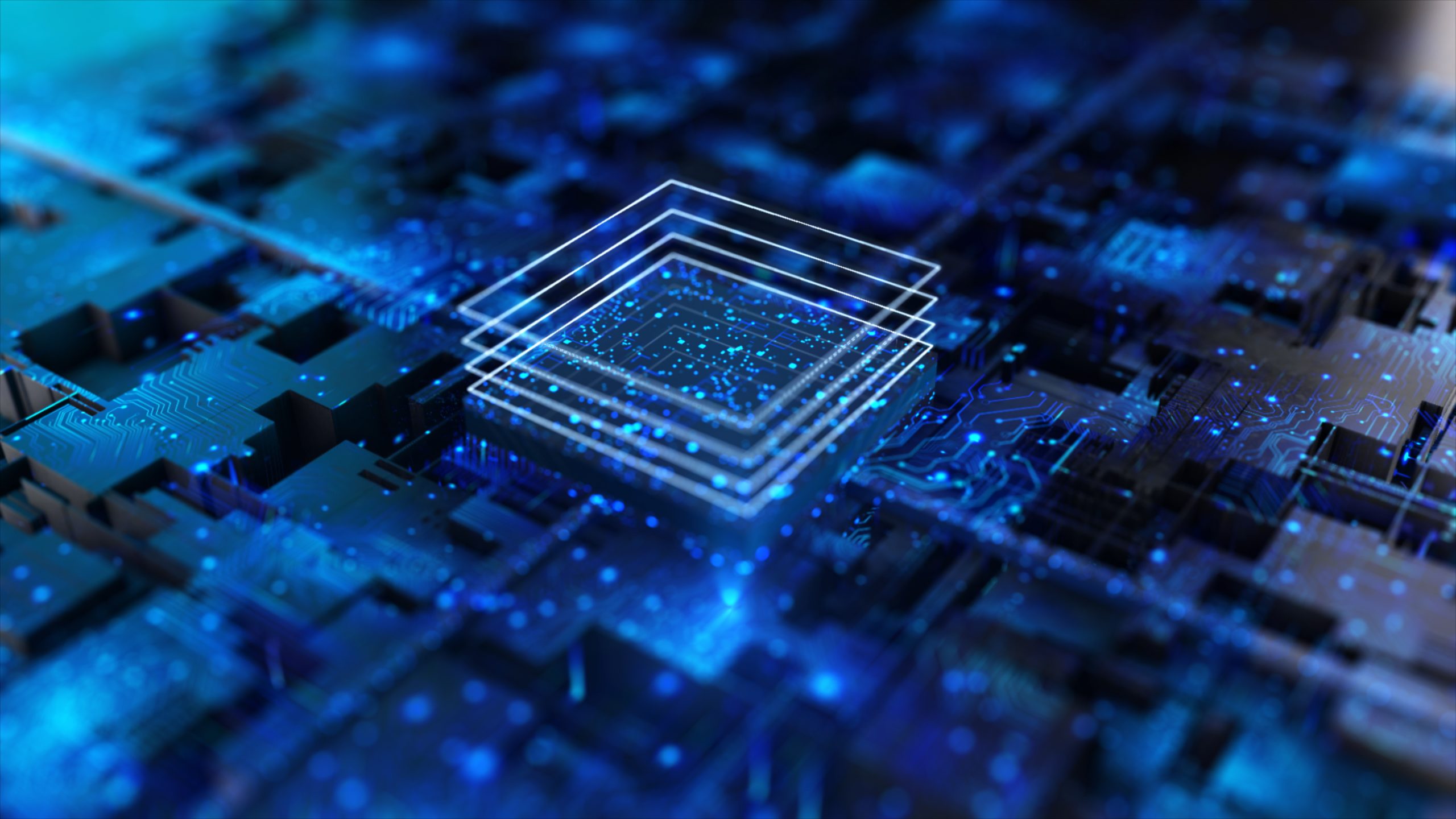
Peter Koo, PhD, assistant professor at the Cold Spring Harbor Laboratory, says that the recent popularity of Artificial Intelligence has led to a shortage of innovation.
It is like the Wild West now. Everyone is doing what they want. Various sources are being used to build new algorithms. It is hard to judge whether their creations are good or bad. How can scientists judge good and bad when dealing with computations that are beyond human capabilities?
GOPHER is a new method created by the lab that helps researchers identify the most efficient programs to analyze the genome. The framework created by the graduate student in the laboratory allows for more systematic comparisons of the algorithms.
Members of the team are working with Peter Koo.
The work evaluating deep learning for predicting Epigenomic profiles was published by the researchers.
Deep learning has been able to predict epigenomic profiles. Most approaches use peak callers to define functional activity for the task. Quantitative models predict the experimental coverage values as a regression. There is a lack of ability to fairly assess the novelty of proposed models and their utility for downstream biological discovery due to the emergence of new models with different architectures and training configurations.
A unified evaluation framework is used to compare various models trained to predict accessibility data. Various modeling choices that affect generalization performance are highlighted. Enhancing model selection and improving variant effect predictions are some of the things that can be improved with the introduction of a robustness metric. Our study supports the idea that quantitative modeling of epigenomic profiles leads to better generalizability.
The method judges the programs on a number of criteria.
How well they learn the biology of our genome, how accurately they predict important patterns and features, their ability to handle background noise, and how interpretable their decisions are, are some of the criteria GOPHER judges artificial intelligence programs on. Tang says that artificial intelligence are the answer to questions for us. She says one of the major issues with them is that they don’t know how they came up with the answers.
GOPHER helped the team dig up the parts of the software that drives reliability, performance, and accuracy. The findings help define the key building blocks for building the most efficient artificial intelligence. Shushan Toneyan, a graduate student at the Koo lab, hopes that this will help people who are new to the field in the future.
Imagine being able to determine what is wrong with you at the push of a button, says Koo. Every doctor’s office could one day be turned into a feature of this science-fiction trope. Similar to video-streaming programs that learn users’ preferences based on their viewing history, artificial intelligence may identify unique features of our genomes that lead to individualized medicine and treatments.
The team hopes GOPHER will help them find the right things for the right reasons, so that they can trust their research. Toneyan says that predictions made for the wrong reasons are not helpful.